Researchers demonstrate how to decode humain brain. Interpreting fMRI to unfold human mind.
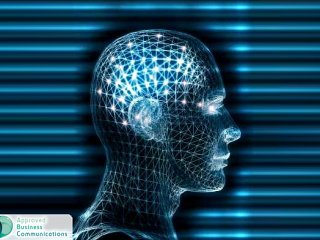
Researchers have shown that it is possible to decode human brains through the use of artificial intelligence. The process, exploiting and scanning fMRI, revels brain functions while watching videos.
The new research may benefit research in the artificial intelligence field as well as improving knowledge over the brain functions. The key asset used is a a type of algorithm called a convolutional neural network, one of the key instruments that allowed electronic devices to recognise face and objects.
“That type of network has made an enormous impact in the field of computer vision in recent years,” said Zhongming Liu, an assistant professor in Purdue University’s Weldon School of Biomedical Engineering and School of Electrical and Computer Engineering. “Our technique uses the neural network to understand what you are seeing.”
In the past, convolutional neural networks, a form of “deep-learning” algorithm, had already been used to study how the brain processes static images and other visual stimuli. However, as the doctoral student Haiguang Wen explained, the new research’s approach represents the first time such algorithm has been used to see how the brain processes movies of natural scenes, representing a step toward decoding the brain while people are trying to make sense of complex and dynamic visual surroundings. The new research paper appeared online on the 20th of October in the journal Cerebral Cortex, with Haiguang Wen as the lead author of the study.
The team acquired 11.5 hours of fMRI data from each of three women subjects watching 972 video clips, including those showing people or animals in action and nature scenes. As first step, the data were used to train the convolutional neural network model to predict the activity in the brain’s visual cortex while the subjects were watching the videos. Then, the researchers used the model to decode fMRI data from the participants to reconstruct the videos, even ones the model had never watched before.
The model managed to accurately decode the fMRI data into specific image categories. Video images were then presented side-by-side with the computer’s interpretation of what the person’s brain had just seen based on fMRI data.
“For example, a water animal, the moon, a turtle, a person, a bird in flight,” Wen explained. “I think what is a unique aspect of this work is that we are doing the decoding nearly in real time, as the subjects are watching the video. We scan the brain every two seconds, and the model rebuilds the visual experience as it occurs.”
The researchers were capable of identifying that certain locations in the brain were associated with specific information a person was seeing.
“Neuroscience is trying to map which parts of the brain are responsible for specific functionality,” Wen added. “This is a landmark goal of neuroscience. I think what we report in this paper moves us closer to achieving that goal. A scene with a car moving in front of a building is dissected into pieces of information by the brain: one location in the brain may represent the car; another location may represent the building.
Using our technique, you may visualize the specific information represented by any brain location, and screen through all the locations in the brain’s visual cortex. By doing that, you can see how the brain divides a visual scene into pieces, and re-assembles the pieces into a full understanding of the visual scene.”
Furthermore, the team was also capable of using models trained with data from one human participant to predict and decode the brain activity of a different participant, in a process defined cross-subject encoding and decoding. Such discovery is significant as it demonstrates the potential for broad applications of such models to investigate brain functions, also for people with visual deficits.
“We think we are entering a new era of machine intelligence and neuroscience where research is focusing on the intersection of these two important fields,” Liu said. “Our mission in general is to advance artificial intelligence using brain-inspired concepts. In turn, we want to use artificial intelligence to help us understand the brain. So, we think this is a good strategy to help advance both fields in a way that otherwise would not be accomplished if we approached them separately.”
Written by: Pietro Paolo Frigenti
Journal Reference: Haiguang Wen, Junxing Shi, Yizhen Zhang, Kun-Han Lu, Jiayue Cao, Zhongming Liu. Neural Encoding and Decoding with Deep Learning for Dynamic Natural Vision. Cerebral Cortex, 2017; 1 DOI: 10.1093/cercor/bhx268