Machine Learning is likely to reshape the job market in the future.
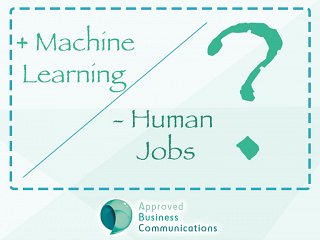
This is suggested by a new Policy Forum commentary written by Carnegie Mellon University’s Tom Mitchell and MIT’s Erik Brynjolfson, published in the December’s edition of the journal Science. Mitchell, who founded the world’s first Machine Learning Department at CMU, and Brynjolfsson, director of the MIT Initiative on the Digital Economy in the Sloan School of Management, describe 21 criteria to evaluate whether a task or a job is amenable to machine learning (ML).
“Although the economic effects of ML are relatively limited today, and we are not facing the imminent ‘end of work’ as is sometimes proclaimed, the implications for the economy and the workforce going forward are profound,” they write.
The new reality will be shaped by the balance between skills people choose to develop and the investments businesses make, which will determine these roles and positions where the human contribution will still be irreplaceable once ML is ingrained in everyday life, they suggest.
ML is one of the elements belonging to the so called artificial intelligence. Rapid advances in ML have recently brought about improvements in natural language understanding, facial recognition and computer vision. Furthermore, ML is already popular for credit card fraud detection, financial market analysis and recommendation systems, with new applications to be expected soon, such as medical diagnosis. Predicting how ML will affect specific roles and professions may be challenging because ML tends to automate, or semi-automate, individual tasks, but jobs often involve multiple tasks, with only some of them replaceable by ML applications.
“We don’t know how all of this will play out,” acknowledged Mitchell, the E. Fredkin University Professor in CMU’s School of Computer Science.
At the beginning of 2017, for example, researchers showed that an ML program could detect skin cancers better than a dermatologist. However, this should not be taken as an evidence that ML will replace dermatologists, as these specialists do many things other than evaluating lesions.
“I think what’s going to happen to dermatologists is they will become better dermatologists and will have more time to spend with patients,” Mitchell observed. “People whose jobs involve human-to-human interaction are going to be more valuable because they can’t be automated.”
Tasks where ML can play a major role can be identified in those with a lot of available data, Mitchell and Brynjolfsson write. In the case of skin cancer detection, for example, ML programs were able to learn through the study of more than 130,000 labeled examples of skin lesions. Similarly, credit card fraud detection programs can learn by analysing hundreds of millions of examples.
ML can play a crucial role in the execution of tasks that already are online, such as scheduling. Jobs where dexterity, physical skills or mobility are not necessary also represent suitable candidates for ML application. Likewise, tasks that involve making quick decisions based on data are suitable for ML programs. However, in such case, the decision should be based on easy reasoning as decisions requiring long chains of reasoning, common sense or diverse background knowledge may not be applicable.
Furthermore, even though ML can be good for specific decisions, as for instance deciding if a lesion is cancerous or not, it may be not adequate to explain how such decision was taken. For such tasks, a dermatologist would be better at explaining the background, the authors suggest. To guess what will be the impact of ML on the market, understanding the possible applications seems to be an essential requirement.
In 2017, a National Academies of Sciences, Engineering and Medicine study on information technology and the workforce, co-chaired by Mitchell and Brynjolfsson, noted that IT progresses have contributed to growing wage inequality.
“Although there are many forces contributing to inequality, such as increased globalization, the potential for large and rapid changes due to ML, in many cases within a decade, suggests that the economic effects may be highly disruptive, creating both winners and losers,” they write. “This will require considerable attention among policy makers, business leaders, technologists and researchers.”
Written by: Pietro Paolo Frigenti
Journal Reference: Brynjolfsson, E. and Mitchell, T. (2017). What can machine learning do Workforce implications. Science, 358 (6370): 1530 DOI: 10.1126/science.aap8062